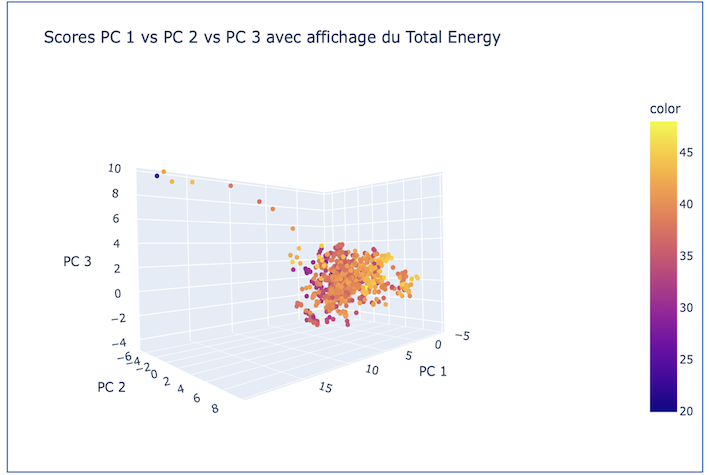
During the month of January, students in the Energy and Processes (ENEP) and Processes and Polymers (POLY) programs, enrolled in continuing education or sandwich-training over 22 months, created predictive models of refining units using machine learning.
Organized in groups of four, the students worked on three real data sets provided by TotalEnergies and two provided by Axens.
They worked on the following topics:
- the prediction of the fuel gas and steam consumption (utilities) of a catalytic reformer unit;
- the energy optimization of a catalytic reformer unit;
- the prediction of the RON (octane number) of the quality of the catalytic reformer charge and
- the energy optimization of a gasoline hydrotreating unit.
This training provided students with a better understanding of how to use available "dense" and/or "possibly inconsistent" data to build a predictive model for qualitative optimization in order to make operations more efficient.
It was also an opportunity to better understand the work of data scientists, to collaborate with experts to build models while ensuring that they follow a correct process.
"For these projects, the students completed three main steps. First, they proceeded to data cleaning, i.e. they cleaned the data by removing marginal elements, isolated points and then they filtered them," explains Stéphane Bourgeon, Supervisor of the Energy and Processes program.
"The students then created a correlation matrix by analyzing the main variables and their impact in the predictive model. Finally, they selected a model from different laws (e.g., linear regression, Ridge, Lasso, etc.)," he adds.